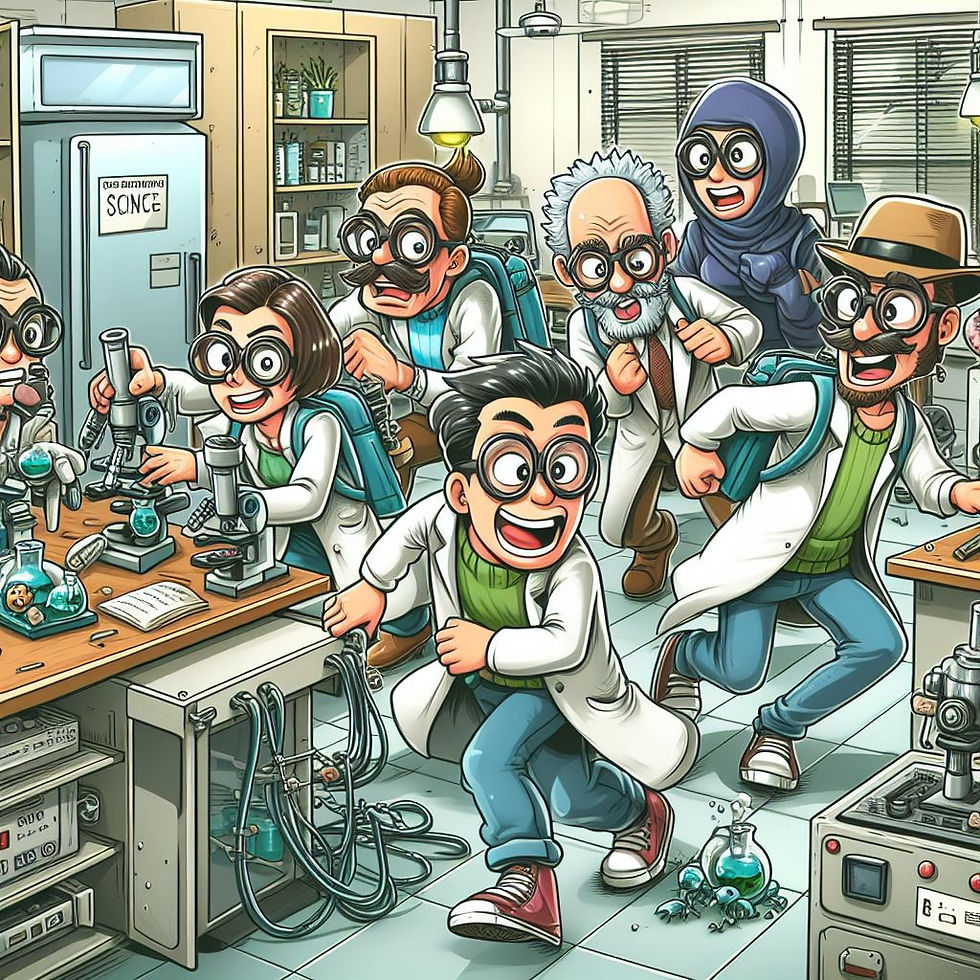
What is Confounding Variable?
Confounding variables, also known as confounders, lurking variables, or extraneous variables, are variables that can distort the true relationship between an independent variable (the one you're studying) and a dependent variable (the outcome you're measuring).
They do this by:
Relating to both the independent and dependent variables.
Not being part of the causal pathway between those variables.
Example:
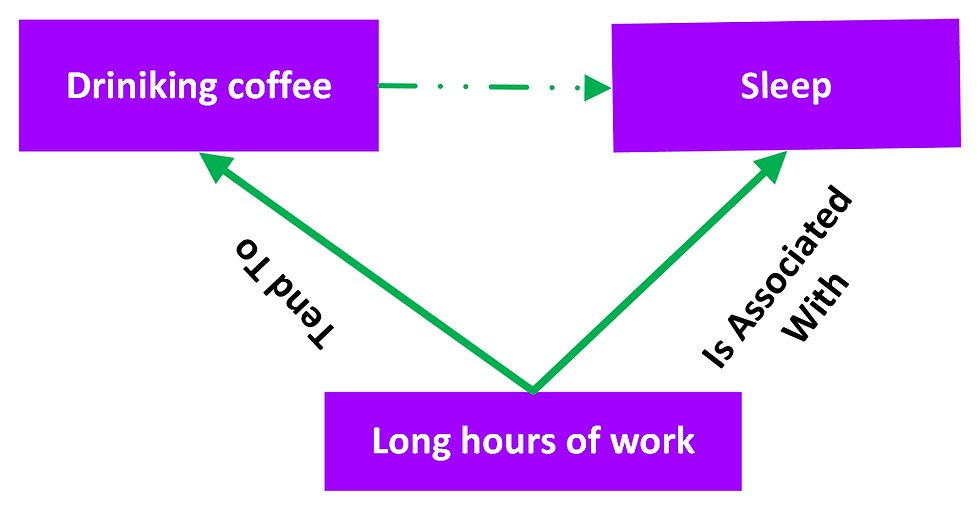
Imagine you're studying whether drinking coffee affects sleep quality. You might find that people who drink more coffee tend to sleep worse.
But what if people who drink more coffee also tend to work longer hours and have more stress, both of which can independently affect sleep? In this case, work hours and stress are confounding variables because they:
Are related to both coffee intake and sleep quality.
Are not part of the direct causal pathway between coffee and sleep.
Types of Confounding Variables:
Selection bias: Arises from how participants are chosen for a study.
Information bias: Arises from how information is collected or measured.
Time-related confounding: Arises when both the independent and dependent variables change over time.
Age-related confounding: Arises when both the independent and dependent variables are related to age.
Controlling Confounding Variables:
Researchers use various methods to control for confounding variables, including:
Randomization: Randomly assigning participants to different groups helps to balance out potential confounders.
Stratification: Dividing participants into groups based on the confounder and analyzing each group separately.
Matching: Matching participants on the confounder to create similar groups.
Statistical control: Adjusting for the confounder using statistical methods.
Importance of Identifying Confounding Variables:
Understanding and controlling for confounding variables is essential in research to ensure that:
Results are accurate and reliable.
Conclusions about cause-and-effect relationships are valid.
Biases are minimized.
Comentarios