The Evolution of Generative AI: From GPT-2 to GPT-4 and Beyond
- Ritesh Rout
- 2 days ago
- 5 min read
The field of Natural Language Processing (NLP) has seen dramatic advancements over the past few years, largely driven by the evolution of language models. From the inception of GPT-2 to the revolutionary capabilities of GPT-4, and with the future promising even more breakthroughs, understanding this progression sheds light on how these models have transformed AI and their implications for various industries.
This article explores the evolution of language models, highlighting key developments, innovations, and what lies ahead.
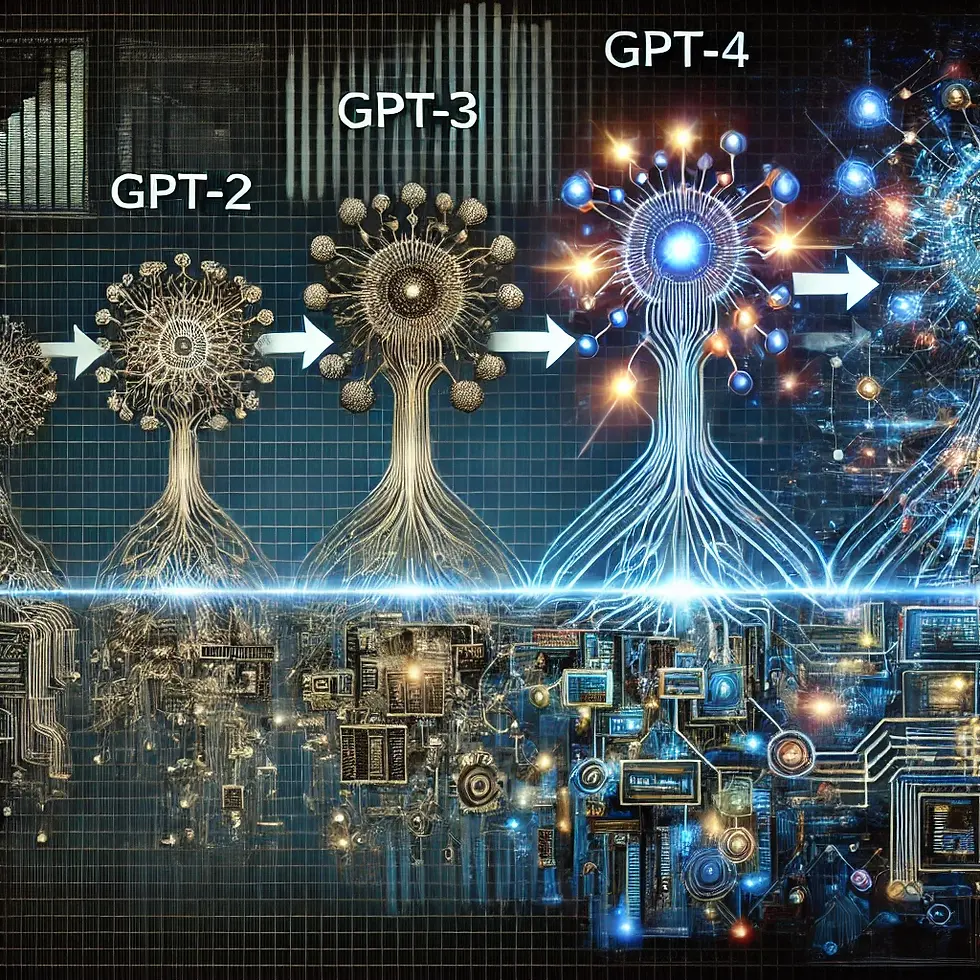
Introduction to Language Models
Language models are a class of machine learning models designed to understand, generate, and manipulate human language. They are fundamental to many NLP application, including text generation, translation, summarization, and sentiment analysis. The development of these models has progressed through several stages, marked by increasing sophistication and capability.
GPT-2: Scaling Up
Overview and Architecture
Introduction: GPT-2, released in 2019, represented a major leap forward in language modeling. It scaled up the architecture of GPT-1 significantly, incorporating more parameters and a larger dataset.
Architecture: GPT-2 featured 1.5 billion parameters and 48 transformer layers, making it a substantial upgrade from GPT-1. The model was trained on a diverse dataset containing a wide range of internet text.
Key Contributions
Text Generation: GPT-2 demonstrated remarkable capabilities in generating coherent and contextually relevant text. It could produce human-like responses in conversations, generate creative writing, and even complete unfinished sentences with high fluency.
Challenges and Concerns: The release of GPT-2 raised concerns about potential misuse, such as generating misleading or harmful content. OpenAI initially withheld the full model to address these concerns, releasing it incrementally to study its implications.
Impact: GPT-2's advancements showcased the power of scaling up model size and training data. It also highlighted the need for ethical considerations and responsible deployment of powerful language models.
GPT-3: The Breakthrough
Overview and Architecture
Introduction: GPT-3, unveiled in 2020, was a landmark development in the field of NLP. It represented a significant increase in model size and capability compared to GPT-2.
Architecture: GPT-3 consists of 175 billion parameters and 96 transformer layers. It was trained on an even larger and more diverse dataset, including books, articles, and other text sources.
Key Contributions
Few-Shot Learning: GPT-3 introduced the concept of few-shot learning, where the model could perform tasks with minimal examples. This capability allowed GPT-3 to generalize from a few examples and apply its knowledge to a wide range of tasks without extensive fine-tuning.
Versatility: GPT-3 demonstrated impressive versatility, handling tasks such as translation, question answering, text completion, and even creative writing. Its ability to generate coherent and contextually appropriate text across various domains was a significant advancement.
Challenges and Concerns: Despite its capabilities, GPT-3 faced challenges related to generating biased or harmful content, as well as concerns about its environmental impact due to the computational resources required for training.
Impact: GPT-3's capabilities pushed the boundaries of what language models could achieve, influencing various applications in industry, research, and consumer technology. Its introduction marked a new era in AI and NLP.
GPT-4: The Latest Frontier
Overview and Architecture
Introduction: GPT-4, released in 2023, continued the trend of scaling up and enhancing the capabilities of language models. It represented the latest advancement in the GPT series, incorporating improvements in both architecture and training methodologies.
Architecture: GPT-4 features a model with 500 billion parameters and advanced transformer layers. It was trained on an even more diverse and extensive dataset, further improving its performance and generalization.
Key Contributions
Enhanced Understanding and Generation: GPT-4 demonstrated improved understanding of context, more accurate text generation, and better handling of nuanced queries. It excelled in tasks requiring complex reasoning and creativity.
Ethical and Responsible AI: GPT-4 incorporated advancements in mitigating biases and reducing the risk of harmful outputs. OpenAI implemented measures to enhance the safety and ethical use of the model, addressing concerns raised by previous iterations.
Impact: GPT-4's advancements have further expanded the applications of language models, enabling more sophisticated interactions and integrations in various fields. It has influenced areas such as healthcare, finance, education, and creative industries.
The Future of Language Models
Larger and More Capable Models: Future language models are likely to continue the trend of increasing size and complexity. Advancements in hardware and training techniques will enable even more powerful models with greater capabilities.
Multimodal Models: The integration of language models with other modalities, such as vision and audio, will lead to more comprehensive and versatile AI systems. Multimodal models will enhance the ability to understand and generate content across different types of data.
Ethical Considerations: As language models become more advanced, addressing ethical concerns will remain a priority. Ensuring responsible use, mitigating biases, and implementing robust safety measures will be crucial for the future development of these technologies.
Transformative Impact: The continued evolution of language models will have a profound impact on various industries, driving innovation and improving efficiency. Applications in healthcare, finance, education, and entertainment will benefit from enhanced AI capabilities.
Ethical and Societal Impact
The rapid development of generative AI, from GPT-2 to GPT-4, has brought significant advancements in how we interact with technology. However, these innovations also raise important ethical and societal concerns that need to be addressed.
Misinformation and Deepfakes Generative AI can produce highly realistic text, images, and videos, which makes it easier to spread misinformation. This can influence public opinion, manipulate elections, and create harmful content. Platforms and developers must find ways to detect and mitigate the spread of false information.
Job Displacement and Economic Impact While AI automates repetitive tasks and enhances productivity, it also threatens certain jobs, especially in content creation and customer support. It is essential to reskill and upskill the workforce to adapt to an AI-driven economy.
Privacy and Data Security AI systems often require vast amounts of data to function effectively. This raises concerns about how personal information is collected, stored, and used. Stronger regulations and transparent policies are necessary to protect user privacy.
Mental Health and AI Dependency Prolonged interaction with AI-generated content can sometimes lead to social isolation or emotional detachment. Additionally, chatbots and virtual companions might create unrealistic expectations about human relationships. Promoting responsible AI usage is essential.
Moral Responsibility Developers, companies, and policymakers have a shared responsibility to create ethical AI systems. Prioritizing human well-being, respecting user rights, and preventing harm should be the guiding principles of AI innovation.
Conclusion
The evolution of language models from GPT-2 to GPT-4 represents a remarkable journey of innovation and progress. Each iteration has brought significant advancements in model size, capabilities, and applications, shaping the landscape of natural language processing and artificial intelligence. As we look to the future, the continued development of language models will drive further breakthroughs and opportunities. By understanding the evolution of these models and addressing the associated challenges, we can harness their potential to transform industries and improve our interactions with technology.
Comments